The ECS-F1HE155K Transformers, like other models based on the Transformer architecture, have made a significant impact across various fields, particularly in natural language processing (NLP) and beyond. Below, we delve deeper into the core functional technologies and application development cases that showcase the effectiveness of Transformers.
Core Functional Technologies of Transformers
1. Self-Attention Mechanism | |
2. Positional Encoding | |
3. Multi-Head Attention | |
4. Feed-Forward Neural Networks | |
5. Layer Normalization and Residual Connections | |
6. Scalability | |
1. Natural Language Processing (NLP) | |
2. Machine Translation | |
3. Question Answering Systems | |
4. Image Processing | |
5. Speech Recognition | |
6. Reinforcement Learning | |
7. Healthcare Applications | |
Application Development Cases
Conclusion
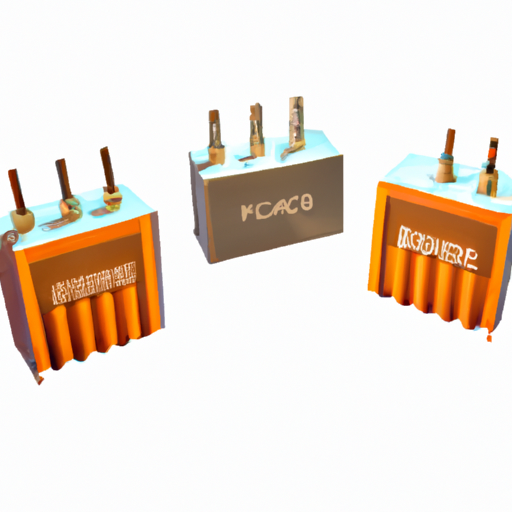
The ECS-F1HE155K Transformers and their foundational technologies have demonstrated remarkable effectiveness across diverse applications. Their ability to process sequential data, capture contextual nuances, and scale effectively has led to significant advancements in fields such as NLP, computer vision, and healthcare. As research and development continue, we can anticipate even more innovative applications and enhancements in Transformer-based models, further solidifying their role as a cornerstone of modern AI technologies.
The ECS-F1HE155K Transformers, like other models based on the Transformer architecture, have made a significant impact across various fields, particularly in natural language processing (NLP) and beyond. Below, we delve deeper into the core functional technologies and application development cases that showcase the effectiveness of Transformers.
Core Functional Technologies of Transformers
1. Self-Attention Mechanism | |
2. Positional Encoding | |
3. Multi-Head Attention | |
4. Feed-Forward Neural Networks | |
5. Layer Normalization and Residual Connections | |
6. Scalability | |
1. Natural Language Processing (NLP) | |
2. Machine Translation | |
3. Question Answering Systems | |
4. Image Processing | |
5. Speech Recognition | |
6. Reinforcement Learning | |
7. Healthcare Applications | |
Application Development Cases
Conclusion
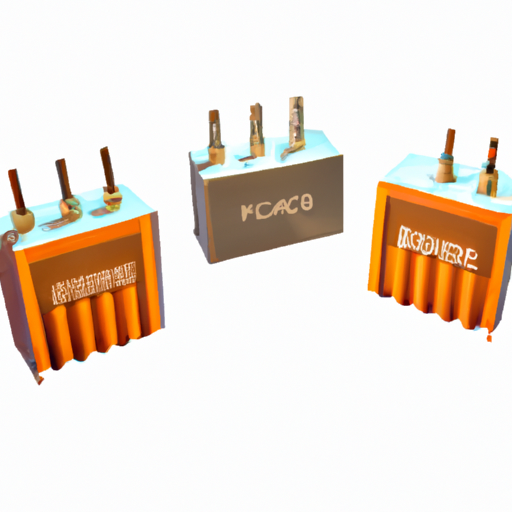
The ECS-F1HE155K Transformers and their foundational technologies have demonstrated remarkable effectiveness across diverse applications. Their ability to process sequential data, capture contextual nuances, and scale effectively has led to significant advancements in fields such as NLP, computer vision, and healthcare. As research and development continue, we can anticipate even more innovative applications and enhancements in Transformer-based models, further solidifying their role as a cornerstone of modern AI technologies.